Project
kliMAGS - Monitoring of climate-impacting measures in crop and grassland management with satellite data
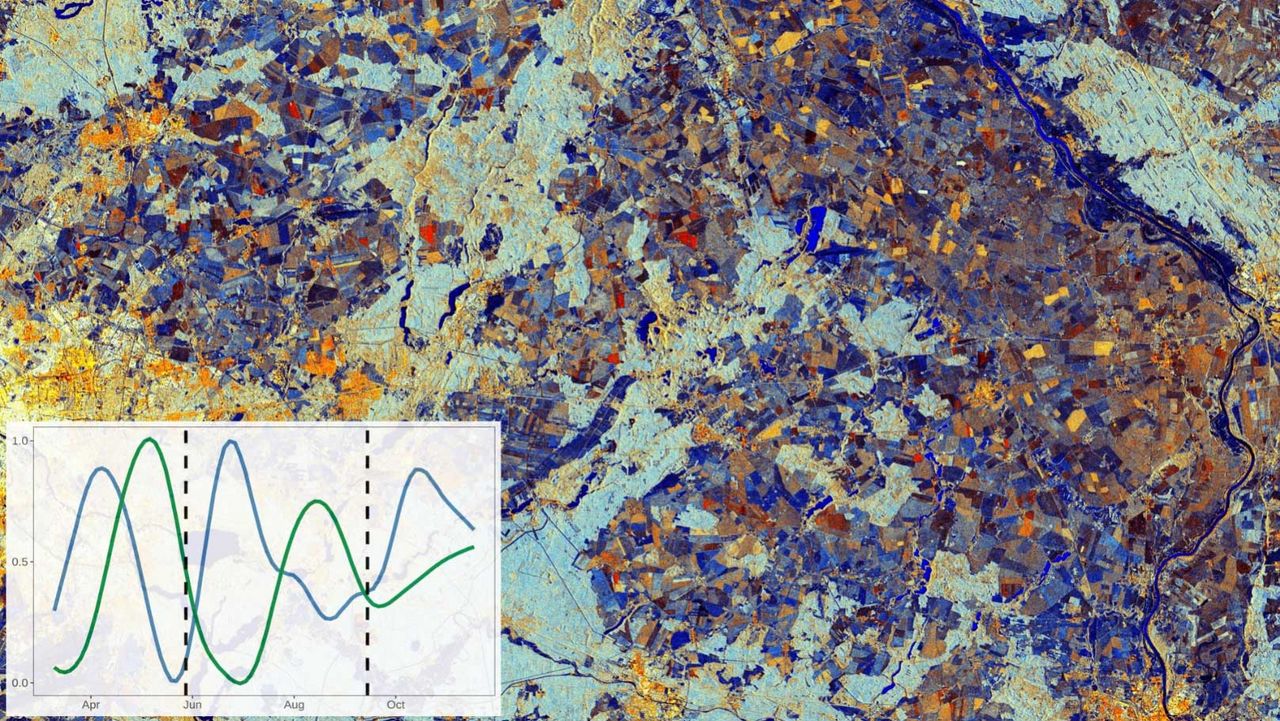
Monitoring of climate-impacting measures in crop and grassland management with satellite data
With 66.4 million tons of CO2 equivalents, agriculture was responsible for 9% of greenhouse gas emissions in Germany in 2020. Adaptations in farm management can reduce these emissions. Area-wide data on such adaptation measures will be derived from high-resolution satellite data.
Background and Objective
With the Climate Protection Plan 2050, Germany has committed itself to become largely climate neutral by the second half of the century. Achieving this goal is not possible without also reducing greenhouse gas emissions in agriculture and contributing to carbon storage and thus to "negative emissions" through appropriate measures.
Environmental and climate protection are increasingly in the focus of the Common Agricultural Policy (CAP) after 2020. According to the current status, at least 25 percent of the direct payments (1st pillar of the CAP) are to be used for environmental and climate protection measures, so-called eco-schemes, and at least 30 percent of the funds of the 2nd pillar are to be used for agri-environmental and climate measures (AECM) for the conservation of resources and the promotion of low-emission and climate-resistant agriculture.
Area-wide monitoring of such services with satellite data enables evaluation of the effectiveness of environmental and climate measures in the agricultural landscape. In addition, remote sensing can provide an essential data basis for improving reporting in the context of achieving the climate change plan targets for the agricultural sector. This includes both long-term developments and short-term fluctuations in emissions-related activities.
The added value of satellite data in this context has been proven, but technical concepts for large-scale, consistent, timely, and reliable implementation are lacking. The project aims to conduct feasibility studies for the operational coverage of climate-related activities from Copernicus satellite data as a contribution to monitoring in the context of CAP and climate reporting.
Approach
Within the scope of the project, methods will be developed to derive the following indicators for climate-impacting measures or activities in agriculture from Copernicus satellite data on an area-wide basis:
Data and Methods
The data of the Sentinel satellites of the European earth observation program Copernicus (Sentinel-1 / -2) with their high spatial and temporal resolution form the data basis for this project. The high repetition rate of the individual Sentinel satellites and their combination to a dense time series enable the area-wide observation of the agricultural landscape at intervals of a few days. Temporal and spatial patterns of the vegetation state, as well as management measures, are mapped and analyzed with the help of machine learning methods.
Our Research Questions
Thünen-Contact

Involved Thünen-Partners
Duration
1.2021 - 3.2024
More Information
Project status:
finished
Publications to the Project
- 0
Lobert F, Löw J, Schwieder M, Gocht A, Schlund M, Hostert P, Erasmi S (2023) A deep learning approach for deriving winter wheat phenology from optical and SAR time series at field level. Remote Sens Environ 298:113800, DOI:10.1016/j.rse.2023.113800
- 1
Lobert F, Röder N, Gocht A, Schwieder M, Erasmi S (2022) Mowing detection from combined Sentinel-1, Sentinel-2, and Landsat 8 time series on fallow cropland with transfer learning. Publikationen der DGPF eV 30:117-126
- 2
Lobert F, Holtgrave A-K, Schwieder M, Pause M, Gocht A, Vogt J, Erasmi S (2021) Detection of mowing events from combined Sentinel-1, Sentinel-2, and Landsat 8 time series with machine learning. Grassl Sci Europe 26:123-125
- 3
Lobert F, Holtgrave A-K, Schwieder M, Pause M, Vogt J, Gocht A, Erasmi S (2021) Mowing event detection in permanent grasslands: Systematic evaluation of input features from Sentinel-1, Sentinel-2, and Landsat 8 time series. Remote Sens Environ 267:112751, DOI:10.1016/j.rse.2021.112751
- 4
Schlund M, Lobert F, Erasmi S (2021) Potential of Sentinel-1 time series data for the estimation of season length in winter wheat phenology. In: Institute of Electrical and Electronics Engineers (ed) IGARSS 2021 - 2021 IEEE International Geoscience and Remote Sensing Symposium : proceedings ; 12-16 July 2021, Virtual Symposium, Brussels, Belgium. IEEE, pp 5917-5920, DOI:10.1109/IGARSS47720.2021.9554454